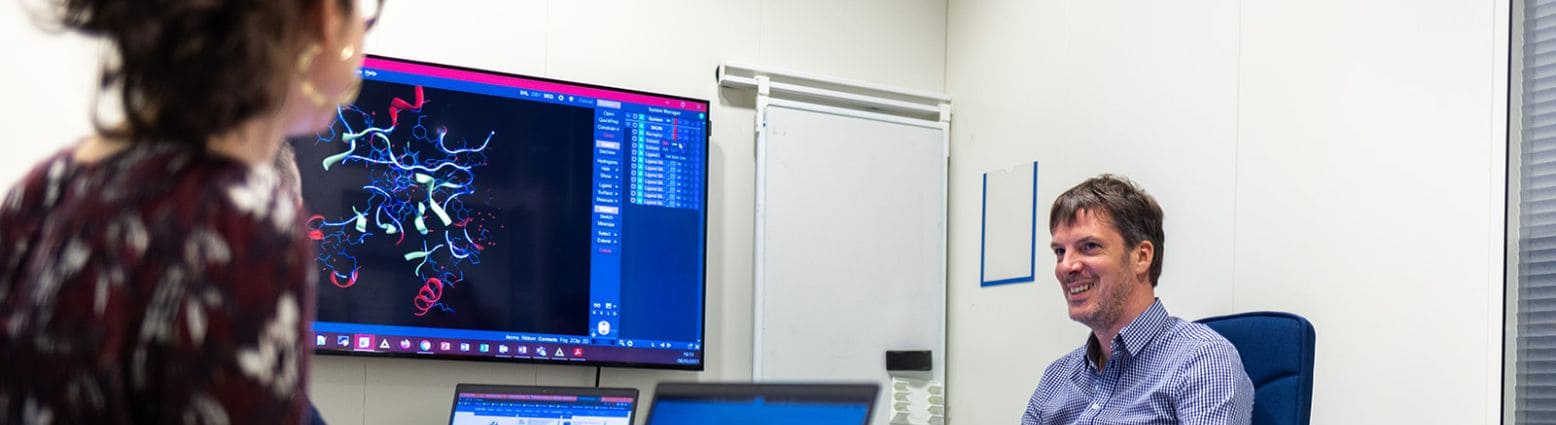
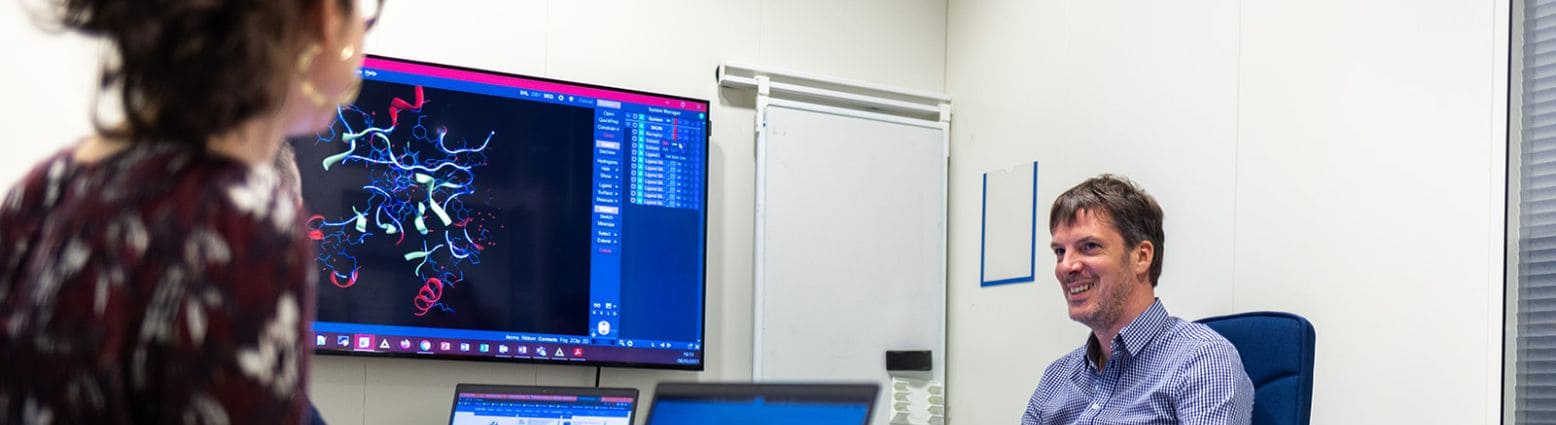
The Game-Changing Role of Artificial Intelligence in Drug Discovery
Historically, Drug Discovery has relied heavily on trial and error, extensive laboratory work, and a considerable amount of serendipity, often taking over a decade and costing billions of dollars to bring a new drug to market1. The integration of artificial intelligence (AI) represents a revolutionary stride in pharmaceutical research. This technological evolution promises not only to accelerate the pace at which new drugs are discovered but also to significantly reduce the costs associated with drug development. The advent of AI in Drug Discovery is poised to change the day to day life of medicinal chemists by introducing computational methods that can predict drug behavior, identify new drug targets, and optimize drug designs with good efficiency.
Here, we explore application of AI throughout the Drug Discovery journey and how it impacts medicinal chemists.
AI’s involvement in drug discovery dates back to the late 20th century, primarily focusing on computational chemistry and bioinformatics. In the 1970s and 1980s, early AI systems were developed to assist in the analysis of chemical compounds2 and biological data3,4. These systems utilized algorithms to predict molecular behavior and interactions, though their capabilities were limited by the computational power and data available at the time. The 1990s saw the introduction of machine learning (ML) techniques in drug discovery5 with significant advancements in computational power and the availability of large datasets. During this period, AI applications in drug discovery were primarily focused on quantitative structure-activity relationship6,7 (QSAR) models, which predict the activity of chemical compounds based on their structural properties. These models provided valuable insights into the molecular mechanisms of drugs and facilitated the identification of potential drug candidates8.
The Evolution of AI in Drug Discovery: from 2000 to now
The early 2000s mark a significant turning point with the rise of more sophisticated machine learning algorithms and the advent of big data. The introduction of high-throughput screening (HTS) technologies generated massive datasets, which AI systems could analyze to identify potential drug candidates more effectively. Furthermore, advances in genomics and proteomics provided a wealth of biological data, allowing AI to delve deeper into the molecular knowledge of diseases and drug actions9,10. By the end of the 2000s, AI had become a very useful tool in drug discovery, aiding in various stages of the drug development process, from target identification to lead optimization. In the past decade, AI has made significant strides in the field of drug discovery, driven by advancements in deep learning, neural networks, and the integration of multi-omics data.
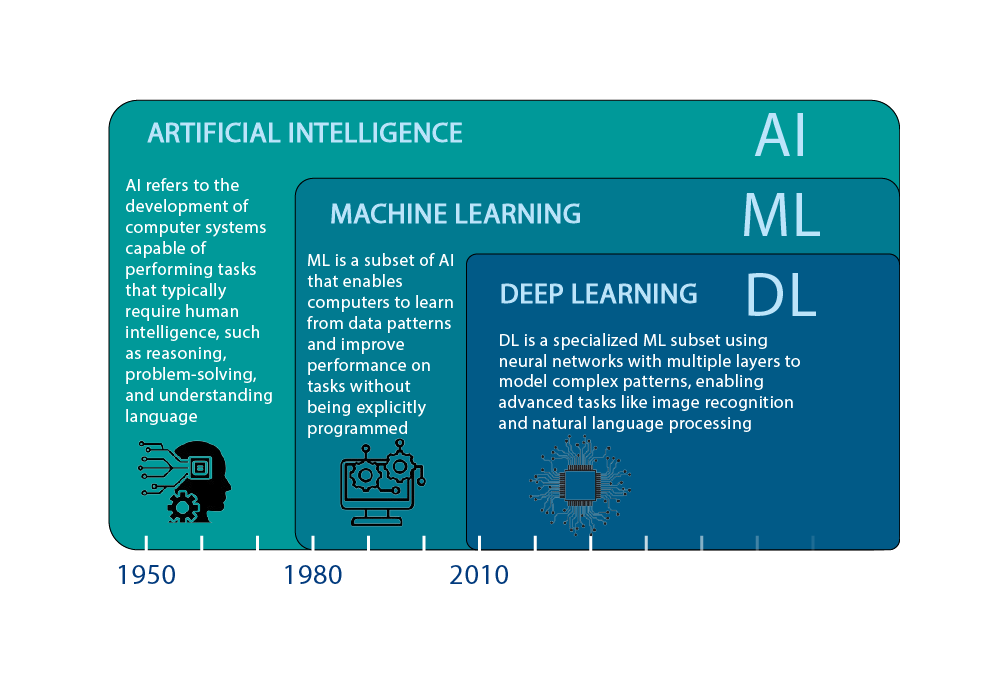
In Target Identification, one of the key areas where AI has shown immense potential, AI algorithms can analyze vast amounts of biological data to identify novel drug targets, which are proteins or genes implicated in disease processes. By using machine learning models to sort through genomic, transcriptomic, and proteomic data, researchers can uncover previously unknown targets that traditional methods might have missed11.
Another critical application of AI is in the prediction of 3D structures of proteins, which is essential for understanding drug-target interactions. The development of AI-based tools such as AlphaFold12,13 or RoseTTaFold14 has revolutionized this field. AlphaFold uses deep learning to predict the 3D structures of proteins with remarkable accuracy, providing invaluable insights into their functions and interactions with potential drugs. This capability accelerates the drug discovery process by enabling more precise modeling of drug-target interactions and guiding the design of molecules with optimal binding properties.
De novo drug design and compound enumeration have also benefited from AI advancements. Generative models, such as variational autoencoders15 (VAEs) and generative adversarial networks16 (GANs), are used to design novel molecules with desired properties from scratch. These models can explore vast chemical spaces and generate new compounds that meet specific criteria, such as high binding affinity or favorable pharmacokinetic profiles. Additionally, AI-driven virtual screening methods allow researchers to rapidly evaluate large libraries of compounds and identify those with the highest potential for further development. AI also plays a role in retrosynthesis prediction17, where Machine Learning models can predict retrosynthetic pathways with a reasonable accuracy, streamlining the synthesis of molecules and reducing the time and cost associated with drug development18. Similarly, AI-driven synthesis accessibility scores19,20 provide insights into the feasibility of synthesizing a given compound, guiding chemists in selecting the most promising candidates for further development.
Furthermore, molecular docking and molecular dynamics simulations have become standard tools in drug discovery. Molecular docking predicts the binding affinity of small molecules to their target proteins21, while molecular dynamics’ simulations provide insights into the dynamic behavior of drug-target interactions over time22. These techniques enable researchers to refine drug designs and optimize binding properties, leading to more effective and selective drugs.
The prediction of biological activity and ADMET (absorption, distribution, metabolism, excretion, and toxicity) properties is another area where AI has made significant contributions. QSAR (quantitative structure-activity relationship) and QSPR (quantitative structure-property relationship) models use machine learning to predict the biological activity and ADMET profiles of compounds based on their chemical structures23. These models help identify potential drug candidates with favorable properties and flag those with undesirable characteristics early in the development process24.
Challenges and Limitations of AI in Drug Discovery
While AI has shown good promises for Drug Discovery, it is important to mention some limitations and challenges that remain. One of the key concerns is the potential for bias in AI algorithms25. If the training data used to develop models is biased, the models will also show bias in their predictions. This can lead to inaccurate results and delay the discovery of effective drugs. To address this issue, researchers are working on developing more diverse and representative datasets and implementing bias mitigation techniques.
Another challenge is the interpretability of the models. Many AI algorithms, particularly deep learning models, are complex and difficult to understand. This can make it challenging to explain how the models come to their predictions, which is important for ensuring trust and transparency in the drug discovery process. Efforts are underway to develop more interpretable AI models and to develop techniques26 for explaining the predictions of complex models.
The integration of AI in drug discovery has transformed the field, offering opportunities to accelerate the development of new therapies while reducing costs. From early applications in computational chemistry to the sophisticated deep learning models of today, AI has become an indispensable tool in the pharmaceutical industry. Current applications of AI, such as target identification, protein structure prediction, de novo drug design, retrosynthesis prediction, and ADMET modeling, have demonstrated significant improvements in the efficiency and accuracy of the drug discovery process27. However, it is important to be aware of the challenges and limitations of AI and not to want to bypass the essential experimental validation stages.
Looking ahead, the future of AI in Drug Discovery is promising
Continued advancements in AI technologies, coupled with the increasing availability of biological and chemical data, will further enhance the capabilities of AI systems. In the following decades, we can expect AI to play an even more integral role in drug discovery, potentially leading to the development of personalized medicines tailored to individual patients’ genetic profiles and health conditions. Moreover, the integration of AI with other emerging technologies, such as quantum computing28,29 and synthetic biology30, could unlock new frontiers in drug discovery and revolutionize the way we develop and deliver therapies.
As AI continues to evolve, ethical considerations and regulatory frameworks31 will also need to adapt to ensure the safe and effective use of these technologies in drug discovery. Addressing issues such as data privacy, algorithmic bias, and transparency will be crucial to gaining public trust and maximizing the benefits of AI in healthcare.
Finally, it is important to recognize that AI is not a substitute for experimental validation. While AI can be a valuable tool for generating hypotheses and prioritizing drug candidates, it is essential to conduct rigorous experimental testing to confirm the efficacy and safety of new drugs. AI should be used as a complementary tool to traditional drug discovery methods, rather than as a replacement.
At Oncodesign Services, our computational chemistry team has deployed many of these techniques to help our clients in their research projects, working in close collaboration with biologists and medicinal chemists. If you would like to know more about how AI is integrated into our CADD platform and how it helps our scientists to rank and prioritize drug candidates within your research projects, please don’t hesitate to contact us.
About the author
This blog post was written by Sylvain Lozano, PhD, computational chemist at Oncodesign Services. In his current role, Sylvain is working closely with the medicinal chemistry team to accelerate drug discovery programs.
-
References
[1] Wouters OJ et al. Estimated Research and Development Investment Needed to Bring a New Medicine to Market, 2009-2018. JAMA. 2020;323(9):844-853.
[2] Robinson AL. Computational chemistry: getting more from a minicomputer. Science. 1976;193(4252):470-2.
[3] Smith DH, et al. BIONET: national computer resource for molecular biology. Nucleic Acids Res. 1986;14(1):17-20.
[4] Masys DR. New Directions in Bioinformatics. J Res Natl Inst Stand Technol. 1989;94(1):59-63.
[5] Bolis G, et al. A machine learning approach to computer-aided molecular design. J Comput Aided Mol Des. 1991;5(6):617-28.
[6] King RD, et al. Structure-activity relationships derived by machine learning: the use of atoms and their bond connectivities to predict mutagenicity by inductive logic programming. Proc Natl Acad Sci U S A. 1996;93(1):438-42.
[7] Mahmoudian M. Quantitative structure-activity relationships (QSARs) of pyrimidine nucleosides as HIV-1 antiviral agents. Pharm Res. 1991;8(1):43-6.
[8] Walters WP et al. Recognizing molecules with drug-like properties. Curr Opin Chem Biol. 1999;3(4):384-7.
[9] Ekins S, et al. In silico pharmacology for drug discovery: methods for virtual ligand screening and profiling. Br J Pharmacol. 2007;152(1):9-20.
[10] Ekins S, et al. In silico pharmacology for drug discovery: applications to targets and beyond. Br J Pharmacol. 2007 Sep;152(1):21-37.
[11] Zhavoronkov A. Artificial Intelligence for Drug Discovery, Biomarker Development, and Generation of Novel Chemistry. Mol Pharm. 2018;15(10):4311-4313.
[12] Jumper J, et al. Highly accurate protein structure prediction with AlphaFold. Nature. 2021; 596(7873):583-589.
[13] Abramson J, et al. Accurate structure prediction of biomolecular interactions with AlphaFold 3. Nature. 2024; 630, 493-500.
[14] Baek M, et al. Accurate prediction of protein structures and interactions using a three-track neural network. Science. 2021;373(6557):871-876.
[15] Bort W, et al. Inverse QSAR: Reversing Descriptor-Driven Prediction Pipeline Using Attention-Based Conditional Variational Autoencoder. J Chem Inf Model. 2022;62(22):5471-5484.
[16] Abbasi M, et al. Designing optimized drug candidates with Generative Adversarial Network. J Cheminform. 2022;14(1):40.
[17] Yinjie Jiang, et al. Artificial Intelligence for Retrosynthesis Prediction, Engineering. 2023; Volume 25, Pages 32-50
[18] Segler MHS, et al. Planning chemical syntheses with deep neural networks and symbolic AI. Nature. 2018; 555(7698):604-610.
[19] Ertl P and Schuffenhauer A. Estimation of synthetic accessibility score of drug-like molecules based on molecular complexity and fragment contributions. J Cheminform. 2009;1(1):8.
[20] Voršilák M, et al. SYBA: Bayesian estimation of synthetic accessibility of organic compounds. J Cheminform. 2020;12(1):35.
[21] McGaughey GB, et al. Comparison of topological, shape, and docking methods in virtual screening. J Chem Inf Model. 2007;47(4):1504-19.
[22] Fukunishi Y and Nakamura H. Statistical estimation of the protein-ligand binding free energy based on direct protein-ligand interaction obtained by molecular dynamics simulation. Pharmaceuticals. 2012;5(10):1064-79.
[23] Heyndrickx W, et al. MELLODDY: Cross-pharma Federated Learning at Unprecedented Scale Unlocks Benefits in QSAR without Compromising Proprietary Information. J Chem Inf Model. 2024;64(7):2331-2344.
[24] Cherkasov A, et al. QSAR modeling: where have you been? Where are you going to? J Med Chem. 2014;57(12):4977-5010.
[25] Ferrara E., Fairness and Bias in Artificial Intelligence: A Brief Survey of Sources, Impacts, and Mitigation Strategies. Sci. 2024; 6(1):3.
[26] Ning Y, et al. Shapley variable importance cloud for interpretable machine learning. Patterns (NY). 2022; 3(4):100452
[27] Ekins S, et al. Exploiting machine learning for end-to-end drug discovery and development. Nat Mater. 2019;18(5):435-441.
[28] Santagati, R. et al. Drug design on quantum computers. Nat. Phys. 2024;20, 549–557.
[29] Robert F. Service, Compound Interest: Practical uses for quantum computers are emerging in chemistry, promising to speed the development of materials, catalysts, and drugs. Science. 2024; Volume 384, Issue 6699, Pages 950-953.
[30] Yan X, et al. Applications of synthetic biology in medical and pharmaceutical fields. Signal Transduct Target Ther. 2023;8(1):199.
[31] Naik N, et al. Legal and Ethical Consideration in Artificial Intelligence in Healthcare: Who Takes Responsibility? Front Surg. 2022; 9:862322.